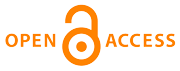 |
Home
>
Journal Issues
>
No 4 (2020) Technical mechanics
>
5
___________________________________________________
UDC 629.5
Technical mechanics, 2020, 4, 43 - 54
Relative control of an underactuated spacecraft using reinforcement learning
DOI:
https://doi.org/10.15407/itm2020.04.043
Khoroshylov S. V., Redka M. O.
Khoroshylov S. V.
Institute of Technical Mechanics of the National Academy of Sciences of Ukraine and the State Space Agency of Ukraine
Redka M. O.
Institute of Technical Mechanics of the National Academy of Sciences of Ukraine and the State Space Agency of Ukraine
The aim of the article is to approximate optimal relative control of an underactuated spacecraft using
reinforcement learning and to study the influence of various factors on the quality of such a solution.
In the course of this study, methods of theoretical mechanics, control theory, stability theory, machine
learning, and computer modeling were used.
The problem of in-plane spacecraft relative control using only control actions applied tangentially
to the orbit is considered. This approach makes it possible to reduce the propellant consumption
of reactive actuators and to simplify the architecture of the control system. However, in some cases,
methods of the classical control theory do not allow one to obtain acceptable results. In this regard,
the possibility of solving this problem by reinforcement learning methods has been investigated, which
allows designers to find control algorithms close to optimal ones as a result of interactions of the
control system with the plant using a reinforcement signal characterizing the quality of control actions.
The well-known quadratic criterion is used as a reinforcement signal, which makes it possible to take into
account both the accuracy requirements and the control costs. A search for control actions based
on reinforcement learning is made using the policy iteration algorithm. This algorithm is implemented
using the actor–critic architecture. Various representations of the actor for control law implementation
and the critic for obtaining value function estimates using neural network approximators are considered.
It is shown that the optimal control approximation accuracy depends on a number of features, namely,
an appropriate structure of the approximators, the neural network parameter updating method, and the
learning algorithm parameters.
The investigated approach makes it possible to solve the considered class of control problems for
controllers of different structures. Moreover, the approach allows the control system to refine
its control algorithms during the spacecraft operation.
relative control, underactuated spacecraft, reinforcement learning, policy iteration, actor–critic
1. MacIsaac D. Docking at the International Space Station. Phys. Teach. 2014. V. 52. No. 126.
https://doi.org/10.1119/1.4862134
2. Campbell M., Fullmer R. R., Hall C. D. The ION-F formation flying experiments. Advances in the Astronautical Sciences. 2000. V. 105. Pp. 135-149.
3. Smith G. W., DeRocher W. L. Jr. Orbital servicing and remotely manned systems. Mechanism and Machine Theory. 1977. V. 12. Pp. 65-76.
https://doi.org/10.1016/0094-114X(77)90058-1
4. Alpatov A. P., Khoroshylov S. V., Maslova A. I. Contactless De-Orbiting of Space Debris by the Ion Beam. Dynamics and Control. - Kyiv: Akademperiodyka, 2019. 170 pp.
https://doi.org/10.15407/akademperiodyka.383.170
5. Vassar R. H., Sherwood R. B. Formationkeeping for a pair of satellites in a circular orbit. Journal of Guidance, Control, and Dynamics. 1985. V. 8(2). Pp. 235-242.
https://doi.org/10.2514/3.19965
6. Redding D. C., Adams N. J., Kubiak E. T. Linear quadratic stationkeeping for the STS orbiter. Charles Stark Draper Laboratory, Cambridge, MA, Kept. CSDL-R-1879, June 1986.
https://doi.org/10.2514/6.1986-2222
7. Dwidar H. R., Owis A. H. Relative motion of formation flying with elliptical reference orbit. International Journal of Advanced Research in Artificial Intelligence. 2013. V. 2(6). Pp. 79-86.
https://doi.org/10.14569/IJARAI.2013.020613
8. Peng H., Zhao J., Wu Z., Zhong W. Optimal periodic controller for formation flying on libration point orbits. Acta Astronautica 2011. V. 69. Pp. 537-550.
https://doi.org/10.1016/j.actaastro.2011.04.020
9. Starin R. S., Yedavalli R. K., Sparks A. G. Spacecraft formation flying maneuvers using linear-quadratic regulation with no radial axis inputs. AIAA Paper. August 2001. Pp. 2001-4029.
https://doi.org/10.2514/6.2001-4029
10. Kumara K. D., Bang H. C., Tahk M. J. Satellite formation flying using along-track thrust. Acta Astronautica. 2007. V. 61(7-8). Pp. 553-564.
https://doi.org/10.1016/j.actaastro.2007.01.069
11. Alpatov A., Khoroshylov S., Bombardelli C., Relative control of an ion beam shepherd satellite using the impulse compensation thruster. Acta Astronautica. 2018. V. 151. Pp. 543-554.
https://doi.org/10.1016/j.actaastro.2018.06.056
12. Khoroshylov S. Relative control of an ion beam shepherd satellite in eccentric orbits. Acta Astronautica. 2020. V. 176. Pp. 89-98.
https://doi.org/10.1016/j.actaastro.2020.06.027
13. Haarnoja T., Zhou A., Abbeel P., Levine S. Soft actor-critic: Off-policy maximum entropy deep reinforcement learning with a stochastic actor. 2018. arXiv preprint arXiv:1801.01290.
14. Jaderberg M., Mnih V., Czarnecki W M., Schaul T., Leibo J. Z., Silver D., Kavukcuoglu K. Reinforcement learning with unsupervised auxiliary tasks. 2016. arXiv preprint arXiv:1611.05397.
15. Khadka S., Tumer K. Evolution-guided policy gradient in reinforcement learning. Advances in Neural Information Processing Systems. 2018. Pp. 1196-1208.
16. Nair A., McGrew B., Andrychowicz M., Zaremba W., Abbeel P. Overcoming exploration in reinforcement learning with demonstrations. 2018 IEEE International Conference on Robotics and Automation (ICRA). 2018. Pp. 6292-6299.
https://doi.org/10.1109/ICRA.2018.8463162
17. Kober J., Bagnell J. A., Peters J. Reinforcement learning in robotics: A survey // International Journal of Robotic Research. 2013. V. 32(11). Pp. 1238-1274.
https://doi.org/10.1177/0278364913495721
18. Khoroshylov S. V., Redka M. O. Intelligent control of spacecraft attitude using reinforcement leaning. Teh. Meh. 2019. No. 4. Pp. 29 - 43. (in Ukrainian).
https://doi.org/10.15407/itm2019.04.029
19. Yamanaka K., Ankersen F. New state transition matrix for relative motion on an arbitrary elliptical orbit. Journal of Guidance, Control, and Dynamics. 2002. V. 25 (1). Pp. 60-66.
https://doi.org/10.2514/2.4875
20. Lewis F. L., Vrabie D., Syrmos V. L. Optimal Control, 3rd Edition. New York: John Wiley & Sons, Inc., 2012.
https://doi.org/10.1002/9781118122631
21. Sutton R. S., Barto A. G. Reinforcement learning: an introduction. MIT Press, 1998. 338 pp.
22. Glorot X., Bengio Y. Understanding the difficulty of training deep feedforward neural networks. Proceedings of the Thirteenth International Conference on Atificial Intelligence and Statistics. 2010. Pp. 249–256.
Copyright (©) 2020 Khoroshylov S. V., Redka M. O.
Copyright © 2014-2020 Technical mechanics
____________________________________________________________________________________________________________________________
|
GUIDE FOR AUTHORS
====================
Open Access Policy
====================
REGULATIONS
on the ethics of publications
====================
|